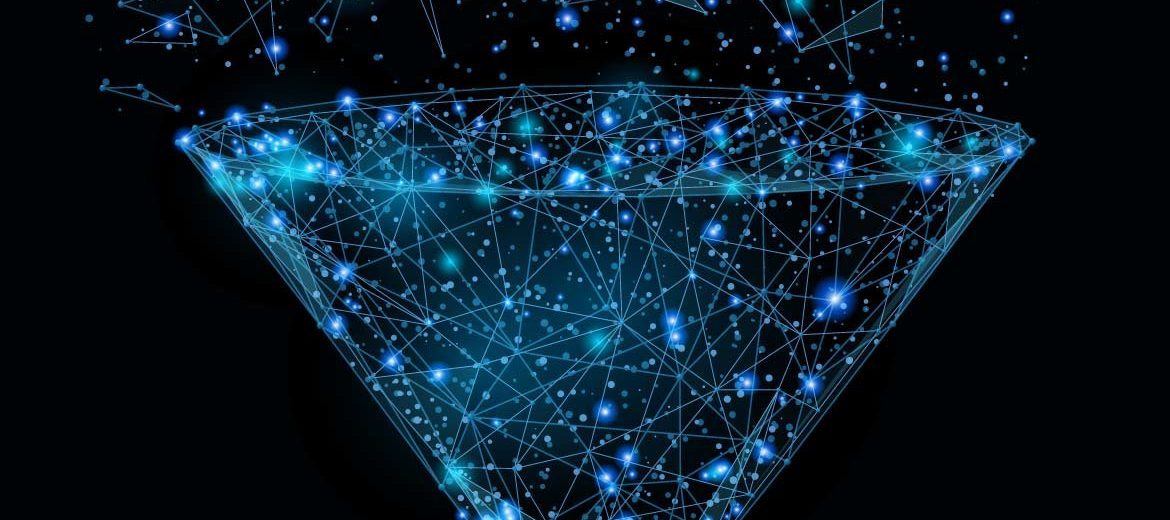
BEFORE REGULATING NEXT-GENERATION MOBILITY DATA, WE MUST UNDERSTAND THAT DATA
The congressional and European Parliament testimonies of Facebook’s CEO focused attention on Internet and ecommerce corporations and startups whose business models rely on the collection and exploitation of big data, with personal data being a major component. Legislators and the public at large came to realize a) the leverage such companies now possess through the dominant positions of the free and frequently personalized services they offer in exchange for the data they collect, b) the risks associated with not properly safeguarding this data, c) the legislators lack of detailed understanding about how the data is collected and used by these companies and their partners, and d) how difficult it will be to regulate the collection, processing, AI-based exploitation, and use of this data in a way that is agreeable to both consumers and businesses. These issues are re-emerging as more connected vehicles are shipped and will become more critical as companies using autonomous vehicles in a variety of services start to employ big data in insights-enabled business models. As we consider the monetization of transportation-related data it is necessary to understand who the main generators and users of this data are, who owns each type of generated data, the risks that may arise from mishandling the collected data, and whether existing and proposed regulations relating to autonomous vehicles and more broadly next-generation mobility suffice or need to be augmented.
Introduction
By 2025 100% of the new vehicles sold worldwide will be equipped with 5G connectivity. In fact, according to a recent report, by 2022 125M vehicles will have Internet connectivity. They will be able to transmit data captured by the vehicle, and receive data from OEMs, automotive suppliers, digital services providers, and the transportation infrastructure. While every vehicle with driving automation of L3 or higher is connected, not every connected vehicle will be autonomous. To appreciate the value of the data generated by connected vehicles today, consider that Toyota recently invested an additional $1B in Grab in order to install sensors in Grab’s cars to collect driving data. Earlier Intel had paid $15B to acquire MobileEye whose ADAS sensors collect data from connected vehicles produced by 27 OEMs. But autonomous vehicles will produce and consume big data of unprecedented detail (impacting volume, variety, and velocity). In terms of volume, consider that when deployed each production autonomous vehicle will be able to produce 4 TB of data per day and Waymo thus far has ordered 82,000 vehicles for its fleets. The value of the next-generation mobility data, including autonomous vehicle data, will be even higher than the data from simply connected vehicles because it will include high fidelity location-specific information, and detailed personal information about the vehicle’s passengers.
In a previous post of this series I had stated that in addition to technology, the deployment of autonomous vehicles under successful business models will depend on a number of different but highly interdependent factors including instituting appropriate regulations. In the United States we are already seeing a variety of regulatory efforts (enacted and proposed) at the federal and state levels regarding the testing and deployment of autonomous vehicles. Similar efforts have started in other parts of the world. However, regulation that relates to the specific characteristics of mobility data, e.g., a consumer’s location at centimeter accuracy, and the inferences that can be made using that data, e.g., a consumer’s health as it can be inferred from preferred transportation modalities, is still lagging. The risks to personal privacy, safety/security, and reputation are high. In fact in many instances they may be higher than those associated with the consumer data collected by Internet, ecommerce, and consumer credit reporting companies (see Equifax’s massive data breach).
Of particular interest is the data utilized by two of the six broad and distinct use cases for autonomous vehicles I had previously identified: on-demand ride-hailing and ridesharing (collectively referred to as on-demand ride services). This is because the companies participating in the value chain of fleet-based on-demand ride services will need to handle big data generated by: a) the vehicles, b) the consumers using these services, and c) their various partners. This data contains important personal information (as one can surmise from Uber’s data breach). It will be used by a variety of important transportation-related applications such as the vehicle’s autonomous navigation, cabin personalization, transportation planning, fleet optimization, and many other important applications. For example, using face recognition technology, the autonomous vehicle can verify that it is carrying the intended passenger and personalize the interior, unlocking certain features. But decisions based on recognition of faces or other body characteristics raise particularly thorny risks. As Apple’s iPhone X demonstrated, your face becomes your password. And passwords are an important target of cyber criminals. Moreover, problems with making wrong decisions using face recognition technologies are already being reported.
Over time we should expect the monetization of mobility-related data collections under novel business models even in use cases that don’t involve transportation. Consider the following: Facebook offers free communication services (Facebook, Messenger, Instagram, WhatsApp). In exchange for offering these free services it is gathering personal data that is organized in a very detailed social graph. The graph’s rich data is now being monetized through advertising and a variety of applications developed by Facebook’s partners. Google achieved a similar result by offering its search engine, email, maps, and other digital services for free. The companies participating in the fleet-based on-demand ride services value chain, particularly the ones offering the actual mobility services, are in the position to create a transportation graph, a structure that organizes peoples’ detailed transportation-related and other personal data, with comprehensive location-based data. We may even envision business models where transportation is offered for free in exchange for such data in order to make the transportation graph broader and more detailed thus increasing its value.
In order to protect individual and corporate rights without stymying new business opportunities for next-generation mobility, it will be important to understand:
- How data from connected vehicles is currently being collected, processed, stored, accessed, and secured, as well as how these practices will change with the introduction of autonomous vehicles. It is important to understand the state-of-practice and state-of-the-art technologies, e.g., blockchain’s ledger, for secure data collection, management, and processing. Regulation based on misunderstood or little-understood technologies, models, etc., is extremely dangerous.
- How consumer mobility data is being exploited today and how it can be exploited in the future using AI and other technologies. Mobility data, i.e., how a consumer moves on a 24×7 basis, has important similarities to the data used in online advertising. For this reason it may be instructive to understand how companies that participate in the online advertising ecosystem use AI technologies to exploit the data they collect in order to effectively market to consumers.
- The difference between a company selling the data it collects and partnering in order to provide access to that data. For example, an automotive OEM that offers Passenger Commerce may not sell the data it captures but may allow access of such data to its commerce partners, e.g., restaurant chains, that can then combine it with their own customer data, and credit card transaction data.
- Future data access rights. It will be important to understand which of the participants in the fleet-based on-demand mobility value chain will have access to the data in the future, and who will have the rights to determine what to do with the data.
- How data-driven business models work today and how such models could transfer to products and services offered using autonomous vehicles. For example, Netflix analyzes consumer data not only to determine what to recommend to its subscribers but also to decide what original content to produce in order to increase its subscriber base and reduce attrition.
- Each regulation’s end-goal: the vehicle’s safe operation to guarantee the consumer’s safety, and the consumer’s protection against various types of risk.
Data generators and data flows
In order to appreciate the risks to consumer privacy, reputation, and safety/security it is important to identify the eight major categories of data generators in the two use cases being considered here:
- Consumers. Because they are using these services.
- Automotive OEMs. Vehicle components such as engine, certain subsystems, tires and other components where it will make sense to place sensors.
- Platform providers. These are the companies providing the AV Operating Platform or the UX Platform. They may be OEMs, Tier 1 suppliers, or startups.
- Fleet leasing companies. These are companies that order and finance the acquisition of vehicles from OEMs and then lease a fleet of vehicles to fleet operators. The companies offering ride services may not have the financial ability to purchase autonomous vehicles outright, so the role of these companies becomes important.
- Fleet operators. The companies offering ride services using such vehicles. As we are also starting to see, in addition to autonomous vehicles, a fleet operator offering ride services may also own and/or have access to fleets of various vehicle types. For example, Uber now owns Jump that operates fleets of dockless bicycles, and recently invested in and is partnering with Lime, a company that operates escooter fleets. Didi and Lyft are following a similar path towards multimodal transportation.
- Fleet managers/maintainers. These companies are responsible for maintaining an operator’s fleet on a daily basis (from refueling/recharging each vehicle, to cleaning it, and repairing it, as appropriate) in order to maximize its uptime and reduce the cost of service.
- Digital services providers. Companies that are providing entertainment, productivity, commerce, mapping, traffic, and other types of digital services.
- Local, state, federal, and national governments. Because they manage certain parts of the transportation infrastructure, including roads and parking.
It is also necessary to understand the data each generator is creating, and how this data is shared among the companies participating in the value chain.
Figure 1 shows the expected data flows between data generators for the ride-hailing and ridesharing use cases. With the exception of consumers who only generate data, the other participants in this value chain have the opportunity to collect and process for their own benefit data that is generated by their partners.
Figure 1: The data flows among data generators
Figure 2 shows in blue some of the data flows that carry privacy risk.
Figure 2: Partial list of data flows that carry privacy risks
A number of different models for operationalizing ride services using fleets that include autonomous vehicles will emerge across the Fleet-Based On-Demand Shared Mobility Value Chain. Each model offers data generators the opportunity to combine the data they create with data they receive from their partners and analyze the resulting databases using AI. For example, Figure 3 depicts the model that will likely be employed by GM’s Cruise division to offer ride services using autonomous vehicles. In addition to manufacturing these vehicles and transferring them from the Chevrolet division to the Cruise division, GM will be a platform provider since they are developing both an AV Operating Platform and a UX Platform, fleet operator, and fleet manager. This means that GM will have direct access to consumer data, vehicle performance data, various types of trip-related data (including video from inside and outside the vehicle), individual vehicle and fleet wide maintenance records, and even digital services-related data (in addition to its OnStar service, GM will offer Passenger Commerce). Under the right partnership agreements, GM will also be able to access data from its government and digital services partners.
Figure 3: GM’s model for operationalizing ride services using autonomous vehicles
Using a different model (shown in blue in Figure 4 below) Waymo will be a platform provider, since they are developing both an AV Operating Platform and a UX Platform that could eventually incorporate lessons and/or components of Android Auto, fleet operator of the 62,000 Pacifica minivans, and 20,000 i-Pace SUVs they have ordered directly from OEMs and which they will make autonomous, and digital services provider (YouTube, Waze, Google Maps). But Waymo won’t be fleet manager (they are partnering with AutoNation and Avis). In addition to the data it generates Waymo by offering consumer ride services, it will also be able to benefit from all the additional consumer data its parent Alphabet has been collecting through its other businesses. For example, the data associated with a consumer’s search for restaurants in a particular location can be utilized in making recommendations to the consumer while being transported. A consumer’s YouTube viewing history can be used in entertainment recommendations during each trip. Finally, Waymo will be able to access the data generated by its OEM, fleet management, digital services, and government partners.
Figure 4: Waymo’s model for operationalizing ride services using AVs
Uber will likely utilize a slightly different model from Waymo’s. Based on this model (shown in green in Figure 5), Uber will be an operator of multimodal transportation fleets that will include at least 24,000 autonomous Volvo SUVsthey have ordered directly from the OEM, dockless bikes, and escooters, and potentially a platform provider (they are developing their own AV Operating Platform and UX Platform while also considering using Waymo’s AV Operating Platform). Similar to Waymo, Uber will augment the data generated by its ride services with the big data from their two-sided ride marketplace. The company will also have access to data generated by its OEM, (platform provider?), fleet management, digital services, and government partners.
Figure 5: Uber’s model for operationalizing ride services using AVs
An example showcasing the data’s implications
To better understand the implications of collecting, augmenting, and analyzing ride services-related data, consider the example below. The example attempts to show the risks associated with the data involved in an activity that will likely become a daily occurrence as autonomous vehicles become part of multimodal consumer transportation options.
As part of the on-demand mobility service provided with an annual subscription, a fleet operator offers to its customers a daily transportation plan. During the sign up process the subscriber is asked to provide: a) transportation preferences from those available in the subscribed tier of service, etc., b) calendar and to-do list access, and c) personal data, including health-related information (that can be used in order to determine, for example, whether bicycle or scooter transportation may be viable options in transportation plans; or whether special vehicles will be needed as would be the case for a handicapped person). During every trip the fleet operator records: a) data from each utilized vehicle’s cabin such as video, audio (for example, passenger interactions with virtual assistants), and passenger-specific biometric data, b) all the data captured by each autonomous vehicle’s outward-facing sensors (data from and about pedestrians, cyclists, points of interest, etc.), and c) the V2X communication data, e.g., data from other autonomous or simply connected vehicles and the transportation infrastructure. We should note that to ensure their drivers’ safety many limo services and taxis in the US already collect in-cabin video and audio data.
Using the provided and recorded data, data from every subscriber’s transportation history, and data provided by its partners, such as expected weather conditions, historic and projected traffic conditions, public transportation loads at times of interest, etc., the fleet operator creates the daily best end-to-end ground transportation plan. There will be several different ways of specifying what is best for a particular subscriber. It may be the cheapest plan, the one that takes the subscriber from one destination to the next in the fastest possible time, or the one that uses the fewest transportation modalities (ride-hailing, ridesharing, walk, escooter, public bus, subway, etc.).
But in the process of creating value for the subscriber, the operator also has the opportunity to record:
- All the places the subscriber visits each day and the order of these visits. In this way the operator can infer the places the subscriber visits routinely.
- Details about each destination. In this way the operator can infer all the businesses operating in a particular building or city block.
- The purpose of each visit. Most of the times this information can be inferred if it is not described explicitly in the subscriber’s calendar.
- The transportation modalities selected by the consumer and how they may differ from those proposed by the operator’s plan. From this data the operator may make inferences about the subscribers health or financial condition. For example, the operator may infer that the subscriber has a medical problem because for a period of several days he did not select transportation options that utilize bikes and escooters, even though he has used such options in the past under fair weather conditions. Combining the transportation modalities used and information about the places the subscriber visits, the operator can infer the subscriber’s financial position.
Such inferences can impact the consumer’s privacy and reputation, as well as expose the consumer to fraud. They will also be of interest to health insurance providers, financial services companies, and obviously cyber criminals.
Therefore, regardless of the model employed by the companies offering ride services using autonomous vehicles, the data and associated AI-based inferences can impact personal a) privacy because they provide detailed understanding about an individual beyond what may be necessary for offering the expected personalized transportation experience, b) reputation because the data and inferences may be incorrect or misused, and c) safety/security because the databases containing the detailed personal data may be breached exposing the subscriber to various types of fraud and even physical danger. As such, this data and associated inferences can be considered as the transportation incarnation of the cookie-based tracking and profiling that websites employ today. But with more profound implications to privacy and physical safety.
Is existing data regulation adequate?
In order to answer this question we must first separate the regulation relating to the safe operation of autonomous vehicles, where we are starting to take certain steps, from regulation relating to the data produced and consumed by connected or autonomous vehicles. In the process, we will need to help governments develop expertise in autonomous systems, and big data technologies. It is also important to understand that in the US federal level, while vehicle safety is the responsibility of NHTSA, data privacy is the responsibility of the FTC. Therefore, the FTC and NHTSA must collaborate in order to address the need for regulation of the data associated with autonomous vehicles and their applications. To this end, it will be important to determine whether:
- Existing data-related regulations suffice or will need to be extended to cover mobility under the business models used and envisioned;
- New regulation will need to be developed from scratch.
With regards to autonomous vehicles the AV START Act is now making its way through the US Senate. This Act is about the development, testing, and safe operation of autonomous vehicles, but doesn’t address data privacy and cybersecurity. In 2014 twenty automakers signed the self-regulating Automotive Privacy Principles. Unfortunately, today this agreement does not cover the data collected by the outward-facing, in-cabin, the data from V2X sensors, payment data used for transportation and Passenger Commerce, or the inferences that can be derived from such data. Similarly, regulation governing the data captured by vehicle Event Data Recorders is inadequate for the data captured by autonomous vehicles.
California recently voted the California Consumer Privacy Act of 2018, a data privacy bill that will go into effect in 2020. When it does, it will allow consumers to opt out of data sharing and prohibit the sale of their data, including on-demand mobility data, to third parties. It is likely that this regulation will be used as a template by several other states that want to enact privacy protections. There are also several other pieces of legislation making their way through Congress including the CONSENT Act that will require consumers to opt-in to share information with technology companies that want to collect and use it, and the SPY Car Act of 2017 that deals with cybersecurity protection of vehicle data, and personal data about driver and passengers. Both of these are stalled and are not expected to be approved any time soon.
The European Union’s General Data Protection Regulation (GDPR) requires corporations to disclose to consumers what data they collect. It provides guidelines on what personal data must be anonymized, including license plates. Later this year or in 2019, the European Union’s ePrivacy Regulation will put in place more rigid requirements for individual consent for the sale and use of customer data in electronic direct marketing. These regulations can be extended to include transportation-related data, as well as face recognition and other personal characteristics that can be utilized by computer vision software to identify an individual. In a piece written Brad Smith, Microsoft’s chief legal officer, he argues that government must regulate facial recognition technologies.
In order to avoid over-regulation it will be necessary for companies in the fleet-based on-demand consumer mobility value chain to embrace a few basic ideas. For example, it will be important to state explicitly and in simple terms what data is necessary to collect for the business model being used to monetize the service offered. Then describe, again using simple terms, what data is actually collected and how it is collected, as well as the types of inferences that are made using this data. This means that if a company collects more data than is necessary for the accomplishment of a stated transportation goal under a specific business model, the user should know it and consent to it. Additionally, the user must have the right to consent to the various types of inferences the company may be making from the data it collects.
Quantifiable value exchange between consumer and service provider must drive these permissions. For example, the owner of a connected vehicle may consent toOver The Air (OTA) updates of a vehicle’s software because such updates are important, thus valuable, to a) the vehicle’s safe operation, and b) introduce new, value-enhancing features. Over time we may need to develop technology that establishes the value for each piece of utilized data and for how long that value holds. The user of the data can then specify why the data may need to be kept for a particular length of time after it is generated and captured.
Obviously, data generators and collectors must also make it easy opting-out from the harvesting of certain types of data even after the provider of the data initially opts-in. For example, a consumer may initially decide to use a free ride service that is monetized through online advertising. In exchange for the free service, the consumer gives permission to the provider to collect personal data. Later on, the consumer decides to switch to a paid subscription of the same service. This means that the form of the value exchange has been modified. Under the paid service the subscriber should be able to opt out of providing as much personal data as under the free service. Some may even say that this should be done automatically without the consumer having to explicitly opt-out.
Ultimately, consumers will need to develop four types of trust with the companies that are part of the fleet-based on-demand shared mobility that use autonomous vehicles:
- Trust that the autonomous vehicle will operate correctly while transporting a consumer to each intended destination.
- Trust that only data necessary for providing the desired mobility services will be collected by the companies involved in the on-demand consumer mobility value chain.
- Trust that the collected data and associated inferences will adhere to established regulations and will be properly safeguarded.
- Trust that the data and the inferences won’t be used in a way that is nefarious and harmful to the consumer.
In considering how to regulate the data associated with autonomous vehicles it is important not to repeat what we are now facing with Facebook and other internet technology companies. These companies created the technology, built business models to monetize the data they collect, and now through regulation governments are trying to clean up the mess associated with personal privacy, reputation, safety, and security. Consumers, corporations, startups, and governments can’t afford to follow the same sequence with autonomous vehicles and next-generation mobility.
Leave a Reply