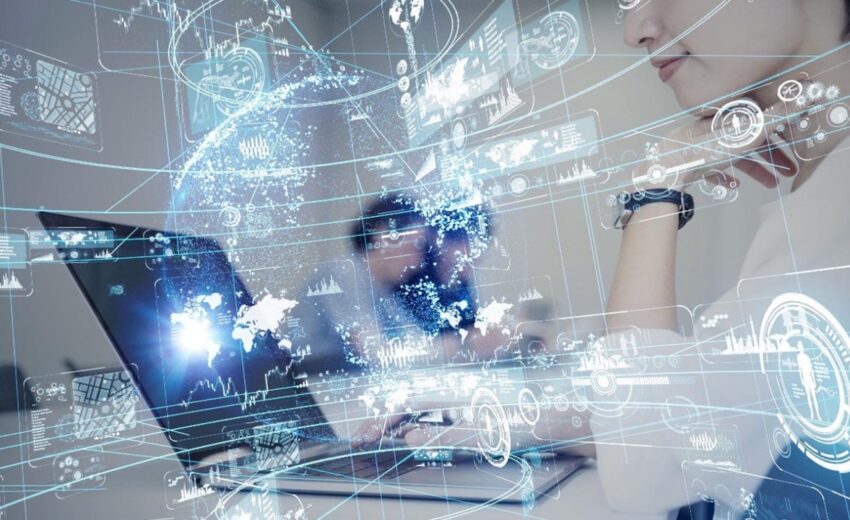
2024 Enterprise AI Themes
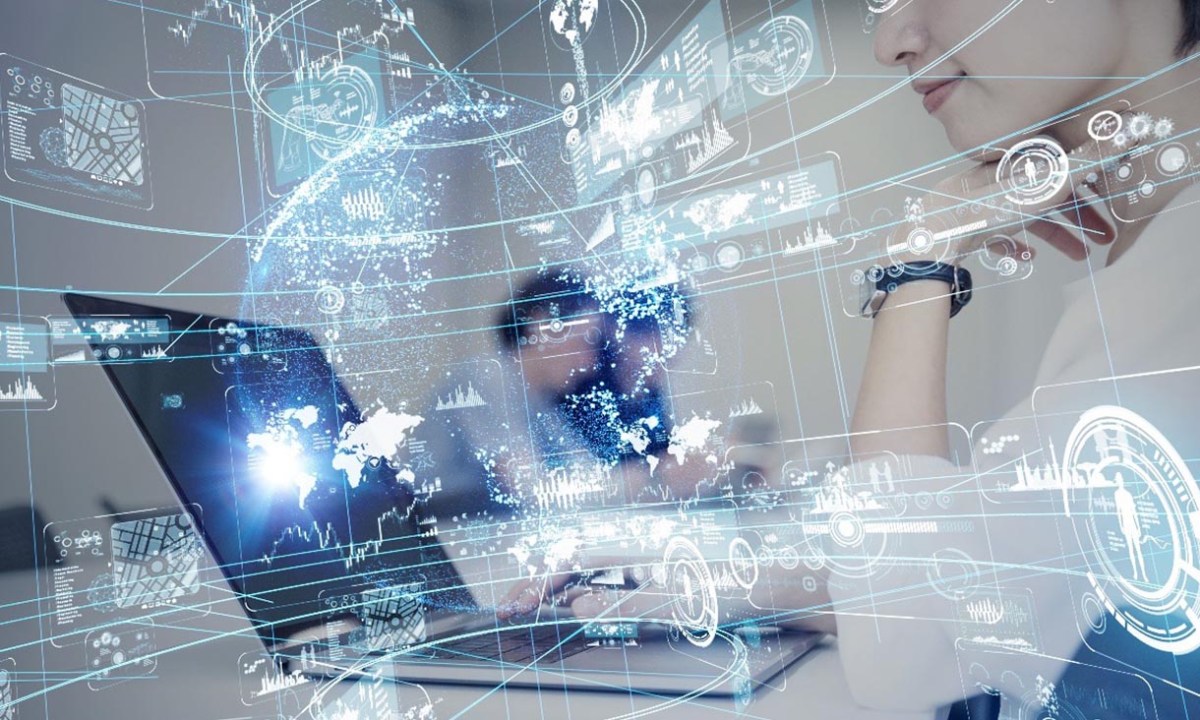
Since the beginning of last year, the interest in AI has gone into overdrive. Enterprises tried to “out-announce” one another regarding their use of AI. Similarly, venture investors have been investing large sums at nosebleed valuations in startups developing anything that appears to involve generative AI. But, despite the corporate statements and the venture investments, the adoption and deployment of AI, and more specifically generative AI by the enterprise, are still in the very early stages.
This is the year during which enterprises from a variety of industries will experiment with discriminative and generative AI. Checks with our firm’s corporate partners allow us to conclude that budgets sufficient for experimentation have already been allocated. Deployment is another matter. The size of these budgets is based on corporate AI strategies that have been devised expeditiously during the last six months. The challenge now is to identify candidate tasks where AI can be applied and specify the forms of value from which each enterprise will benefit so that successful experiments can lead to deployments. Three big themes will emerge around the experimentation: task selection with the associated value drivers, data provenance, security and governance, and AI regulation.
Task Selection
In selecting the tasks on which to experiment using AI, enterprises will have to think about the following four possibilities:
- Tasks that have been previously automated using non-AI methods but where the incorporation of AI can result in important benefits. An example task is financial report generation (data integration, statement generation, and audit). This task has already been automated using ERP systems. However, the use of AI systems will enable more extensive data integration and automatic error correction, better planning, and forecasting, as well as automate the generation of the statements and their auditing.
- Tasks that have only been partially automated, but can now be fully automated with the use of AI. A good example of one such task can be found in automotive manufacturing. Automakers today use numerically controlled (NC) robots in their assembly lines. With these robots, they automate parts of the vehicle assembly process, but a significant number of workers are still required. The replacement of NC robots with AI-based robots, that can plan and operate autonomously adapting to the assembly line conditions as necessary, combined with the broader adoption of New Energy vehicles that have fewer parts than internal combustion engine vehicles and new manufacturing technologies such as gigapressing, will lead to the primarily automated assembly lines and require a few supervising workers.
- Novel tasks that are emerging because of recent AI advances such as personalized learning, personalized precision medicine, and others. As the technology that employees in every industry must utilize becomes more complex and new processes are introduced, the importance of personalized learning is increasing. Consider, for example, the need to train the automaker’s assembly line employees how to collaborate with autonomous robots, or a bank’s programmers how to utilize programming copilots. Personalized employee training involves creating a curriculum that is specific to the employee’s needs. The AI system must understand the employee’s background and learning abilities, design and assemble the instruction material to address them, deliver the material at the appropriate pace to suit the employee, assess the employee’s performance, and adapt the instruction as necessary.
- Tasks that already incorporate AI, e.g., customer support, or search, that can improve exponentially by adding LLMs. Over the years, AI has already transformed customer support with the use of early versions of chatbots. Similarly, search uses AI to, among other functions, predict user intent. Generative AI will lead to the development of personalized intelligent chatbots for customer support, and result in search engines that interact with their users through natural language and enable personalized, multimodal, and contextual experiences.
Successful experimentation in any of these four areas will lead to changes in the nature of work, blue- and white-collar, with both positive and negative consequences. Scaling for experiments to deployments will necessitate corporations to not only determine their LLM strategies but more broadly decide how to approach the emerging generative AI technology stack, including which of its components to bring in-house and for which to rely on suppliers and partners.
Data
The realization that data is the key ingredient to building increasingly sophisticated AI systems for a broadening set of tasks makes data provenance, security, and governance a key theme for 2024.
The use of generative AI for content creation, from letters to marketing copy, and computer programs is leading corporations to start asking about the provenance of the data used to train the LLMs their employees are using. Content creators, like the NY Times, Getty Images, and others, want to protect their copyrighted work from being used without their permission to train LLMs. The majority that are experimenting with LLMs are worried about creating content that is based on unlicensed data, data of questionable provenance, as well as data that includes various biases.
Understanding the data’s provenance is also important because it has security implications for the publicly available models being developed and constantly enhanced. “Contaminated” data that is introduced into a data set by a business or national adversary, or a criminal group, can result in a variety of cybersecurity threats, damage a person’s or an organization’s reputation, or lead to undesirable and uncalled-for actions.
As AI is used by a growing group of employees, both for building and fine-tuning various models, the importance of data governance is increasing. Data governance must now consider a variety of users from the employee who creates simple prompts, to the data scientist who builds LLMs, the application developer who accesses such models through APIs, the security team, the compliance team, the CFO’s team that assesses the costs associated with training, updating, and inference costs, and maybe several others. Governance must include both the training phase of model development and the inference/reasoning phase of model and other organizational knowledge usage.
Regulation
Though excited about the opportunities it ushers, consumers, corporations, and governments are worried about the risks AI raises. The broad availability of open-source and proprietary LLMs makes these risks imminent rather than hypothetical. We are already seeing how generative AI is being used in deepfakes and phishing. The need for regulation is becoming evident to most, though, as I stated before, countries are taking different approaches to it. In the US I expect limited federal action. President Biden’s executive order may provide some direction but requires a level of activity by the various Departments that the federal government has not been known for. The US Congress will continue to proceed with the formation of various working groups that will study issues but not produce something concrete. I am more hopeful that we will see more results at the state level. States such as California, New York, Texas, Florida, and Massachusetts can lead the way. As I described in a previous post, the EU and China have taken different approaches to AI regulation with the EU’s efforts being the most comprehensive to date.
Hard work needs to be done to determine exactly the type of regulation that is needed which implies that the regulators must first understand the technology and its implications on an industry-by-industry basis. Consumers are concerned about accuracy, biases, security, safety, and privacy. Corporate concerns are the same but also extend to IP protection.
We entered 2024 with the activity around AI continuing at a high pace. Technical developments are announced daily. Enterprises are monitoring these developments but need to ensure that they keep abreast of these developments and focus their AI efforts on the areas that will enable them to maximize their returns at risk levels they can tolerate, and with budgets they can afford.
Leave a Reply