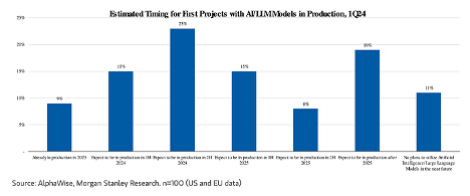
Where Are We With Enterprise Generative AI?
Despite the noise from high-tech corporations, startups, venture investors, and analysts, we are still in a very early phase of generative AI. As a result, it is hard to assume, let alone declare, that generative AI will transform every business process driving operational efficiencies, create new revenue streams, result in massive productivity improvements, and curtail costs. However, the early signs are adequately encouraging for corporations to allocate budgets and start pilot projects.
Corporations are investing in AI. Fourteen percent of CIOs surveyed by Morgan Stanley identified AI as a top corporate priority. Goldman Sachs reports that for this year three percent of corporate IT budgets will be allocated to AI projects, a number which the firm expects to double within three years. In most corporations today the AI investment amounts remain small but critical, nonetheless.
These projections are important for two reasons. First, they follow the pattern that we saw with the enterprise’s adoption of cloud computing. This implies that as AI applications demonstrate value, we can expect the allocated budgets to increase as it happened with cloud computing budgets over the years. Second, we’re still in the early innings of enterprise adoption of generative AI. This phase of the current AI spring is driven by individuals experimenting with broadly available AI applications, mostly chatbots such as ChatGPT, and Large Language Models (LLMs), often at home, rather than in response to a corporate mandate. As they realize benefits from their private use of AI technologies, enterprise employees will drive their adoption in their companies around relevant use cases. We saw this pattern from individual to enterprise-wide adoption with email (remember how AOL email drove the adoption of corporate Internet-based email systems) and cloud computing (individual credit card subscriptions to Salesforce’s sales management application drove the adoption of other cloud-based enterprise applications).
However, it will take time before the pilot projects starting this year can go into production. According to a Morgan Stanley survey, eighty-nine percent of the surveyed CIOs expect to deploy generative AI projects shortly. This number represents a marked increase over the 69% reported by a survey conducted by that firm at the end of 3Q23. Of this eighty-nine percent, 9% of CIOs already had AI projects (including generative AI) in production in 2023, 38% expect such projects to be in production during 2024 (most likely during the latter part of the year), while 42% of CIOs expect to be in production in 2025 and beyond (see figure below).
Another cut of that data shows why the number of corporations starting generative AI pilot projects continues to increase quarterly. As shown in the figure below, as time passes more corporations are becoming convinced that generative AI will significantly impact their operations.
Through these pilot projects, corporations explore several use cases to determine the value generative AI, i.e., applications, LLMs, and infrastructures, adds. Our firm’s efforts with corporations from various industries and general inquiries we receive show that customer support, various marketing tasks, and software programming assistance are the dominant use cases across industries. However, we are also aware of more unique use cases, such as drug discovery, new material discovery, and the identification and replacement of PFAS chemicals from existing products, where generative AI provides unique value. Data availability, quality, rights, security, and privacy considerations remain obstacles to many of these pilots and the broader deployment of the use cases that will be viewed as successful.
For their AI pilot projects most enterprises today use available LLMs without any modification. Most frequently they gravitate to the better-known foundation models rather than exploring which of the large number of available LLMs will best fit the use case they pursue. However, regardless of the model being used, they recognize that LLM response accuracy, hallucinations, and explainability remain critical issues to be addressed. As the data below shows corporations are already experimenting with model fine-tuning and Retrieval-Augmented Generation (RAG).
We are aware of, and are involved in, several efforts to address LLM shortcomings. Some of these efforts focus on improving corporate data quality and orchestration. Others focus on improving LLM performance using model parameter modification, instruction-based prompting, and instruction-based learning. Even though RAG shows promise in pilots, it still requires further experimentation before its value to production systems can be established. Each of these approaches to addressing LLM shortcomings makes different assumptions and imposes unique requirements on the data that can be used and the extent of the changes the LLM can accommodate. Corporations do not yet understand these requirements and their potential impact on production systems.
The costs (initial and ongoing) associated with generative AI projects can be significant. We have yet to see the deployment costs associated with any of the use cases currently in production. Identifying the right use case and analyzing its economics will be one of the key selection criteria.
Even though LLMs are the main components of today’s generative AI pilots, other technologies are also employed. These technologies, however, are still in flux. In some cases, enterprises utilize the complete AI stacks offered by the hyperscalers (from storage and computing to LLMs). A second group develops prototypes using application providers (Adobe, ServiceNow, Salesforce, Workday), and a third uses data management vendors (Snowflake, and Databricks). With small projects, it may even be possible to run these pilots using the facilities of the corporate IT centers. Over time we expect that a meaningful number of AI systems will be deployed through hyperscalers as corporations realize that generative AI requires specialized data center architectures and other resources that their data centers don’t have and will be too difficult and expensive to acquire.
Where does this leave us?
Corporations must continue to experiment with generative AI by utilizing their corporate data and a variety of publicly available, but not necessarily open-source, LLMs. In the process of conducting pilot projects, they must determine whether the use case they pursue necessitates the development of proprietary models or fine-tuning existing LLMs with their proprietary corporate data. If they start with an existing LLM they must make an effort to match the use case with the model’s overall abilities and determine the conditions under which their data will become available for model fine-tuning.
Corporations must prioritize looking for opportunities to reduce model training and inferencing costs by understanding the impact of the data used and the LLM(s) utilized, the cost of their own IT resources, as well as the costs associated with moving workloads to the right outside infrastructure provider be it a hyperscaler, an application provider, a data management provider.
Finally, corporate AI strategies must establish the criteria for moving from pilot to production and include the deployment plans for each system that will be further developed and broadly deployed. The focus should be on identifying a small number of use cases (1-3) that satisfy the established criteria and can be supported by the already allocated and projected budgets over several years rather than just a few quarters. Underfunding such projects will not ultimately enable the corporation to recognize their full potential.
Leave a Reply