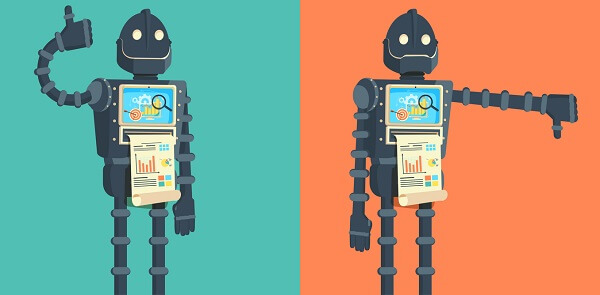
The Struggles Enterprises Face with Generative AI
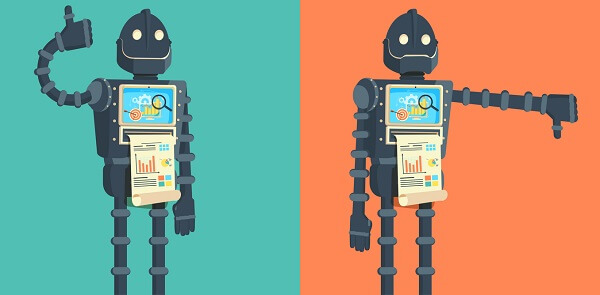
A few days ago I attended the strategy meeting of a portfolio company. Like all of our Synapse Partners portfolio companies, this one provides AI solutions to enterprise customers. Their initial success is in the medical devices industry. While reviewing the company’s sales pipeline and the progress of delivering the company’s solution to signed customers, several of the enterprise’s struggles with generative AI became evident. These struggles can be attributed to a lack of people with the right background and the state of the enterprise data.
Survey after survey across industries makes clear that enterprises are involved in an expedited effort to determine how generative AI can fit into their existing business processes and enable new ones. They are formulating strategies, considering which of their processes are good candidates for AI-based automation, and testing various of the new tools developed for generative AI. This process is harder than most imagined. It is not only because generative AI as a field is moving incredibly fast. But because in most cases the enterprises, as well as the startups, do not have the right people, or the right number of people, to undertake the necessary work.
The issue of people is more severe than I had originally considered. Thanks to the massive hiring drives of the big technology companies, and the lack of attention by enterprises in other industries to protect their AI experts, enterprises find themselves with understaffed AI organizations. As a result, enterprises have to rely on outside consultants for strategy formulation, their proof of concept (POC) projects move slower than expected, some don’t move at all, and conduct fewer AI experiments than they originally planned. In several instances, POCs are left incomplete. This implies that AI application deployment and associated budgeting decisions, which many corporations claim they will make in the October-November timeframe, will be based on incomplete information. Starting deployments with poorly staffed organizations increases the probability of the AI project’s failure.
Enterprises experimenting with generative AI typically take one of two approaches. They develop a chatbot using their proprietary data in conjunction with either a foundation model, such as GPT-4, Gemini, Claude, and others, or an application, such as the one offered by our portfolio company, which incorporates an AI model. Alternatively, they try to develop their own generative AI application, as JP Morgan, Wells Fargo, Experian, Walmart, United Health, Sony, and a few other corporations are trying to do, or have already done. Regardless of the approach, the enterprise’s proprietary data is key to the effort’s success. For this reason, the state of the enterprise’s data is extremely important. I heard from our portfolio company, but also from some of our firm’s advisors, that the data enterprises used to fine-tune their LLM left a lot to be desired in terms of quality, and format. Our portfolio company’s services people had to deal with handwritten notes that needed to first be digitized, the handwriting interpreted and then analyzed by experts to fill in missing context, before each record could be properly labeled and presented to the fine-tuning process. This surprised me. I thought that after the work that enterprises undertook for their business intelligence initiatives over the past twenty-plus years, their data would be in a better condition. Maybe it is because generative AI is enabling corporations to utilize data that business intelligence never did.
In addition to the quality of their proprietary data, enterprises must address data integrity, governance, and ethics to develop generative AI models. Data integrity, governance, and ethics encompass the need to maintain trustworthy, unbiased, safe, and legally compliant datasets, all of which are critical when fine-tuning LLMs. Enterprises need to:
- Handle data ethically to ensure that models are not perpetuating harmful stereotypes or systemic biases. Doing so addresses each decision made by the model with fairness and responsibility.
- Ensure and verify that the data does not lead to unsafe outcomes/decisions and harmful content when the generative AI model is deployed.
- Remove data that has been intentionally or unintentionally corrupted or compromised. Such data could lead to security breaches and negatively impact the reliability of the model. Anthropic is aggressively working in this area.
- Safeguard privacy as it relates to both the ethical and legal aspects of using data that may contain personal or sensitive information. Ensuring compliance with regulations like GDPR or CCPA is essential, and goes beyond just securing the data.
- Guarantee the provenance of the data used to create the underlying model before starting the fine-tuning process to avoid litigation, cybersecurity, and other risks.
Corporations are starting to realize that even a medium-sized generative AI experiment requires the use of several hundred thousand, or even low millions, of proprietary documents to fine-tune a model. Such a document corpus will need to be preprocessed to address the issues listed above. Once this is done, the resulting corpus will need to be properly labeled. The model fine-tuning process can only start after these steps have been completed. So, even if a step such as tokenization of a large document corpus is cheap and getting cheaper, the steps before that can be very expensive depending on the size, quality, contents, and complexity of the data used.
The activity reported by our portfolio companies, our firm’s corporate advisory efforts, but also various corporations through their quarterly reports lead us to conclude that the enterprise’s AI experimentation is robust and accelerating. This is extremely encouraging. We believe that the broad and fast personal adoption of generative AI chatbots initially to accomplish personal tasks and later to perform certain work-related tasks is driving enterprises to explore how to benefit from these technologies. The struggles enterprises encounter during this phase, which we and our portfolio companies help them address, are not surprising given how unprepared enterprises were for AI and how fast generative AI is advancing. The expectation is that enterprises will climb the technology’s learning curve, will not stop their efforts when we enter the trough of disillusionment and continue to address their data issues, allocate the appropriate budgets for successful experiments, and realize the ROI that will cause them to move forward with the broader application of generative AI on their business processes.
Leave a Reply