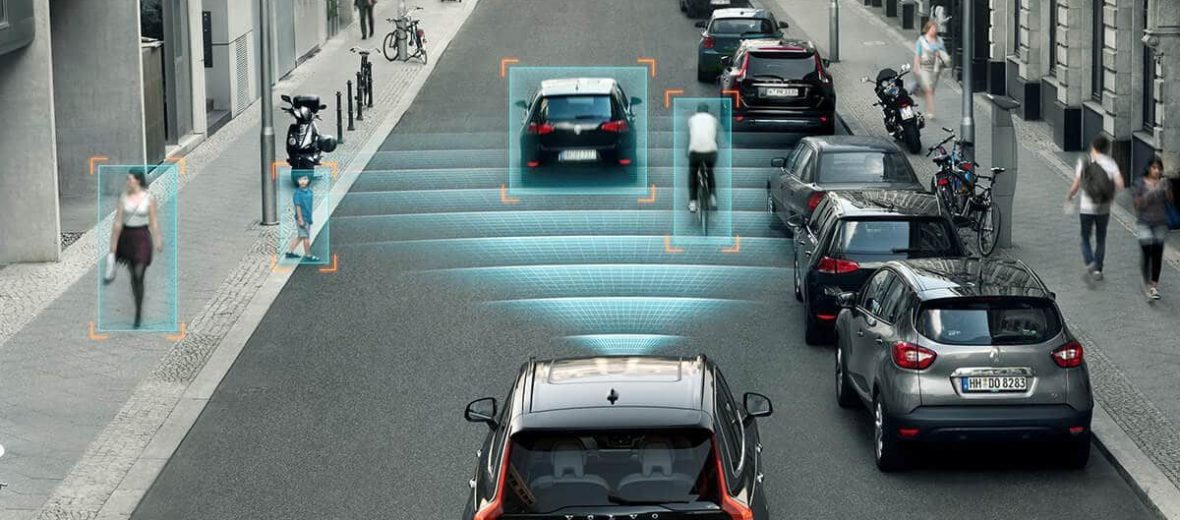
Requirements for Growing and Scaling Autonomous Mobility
The driverless future promised by next-generation mobility will be made possible by two innovations: on-demand mobility services, e.g., ride-hailing, last-mile delivery, and autonomous vehicles used for consumer transportation and logistics. An innovation, including technology innovations, and its adoption advance along a four-phase lifecycle. Today we can evaluate the performance of the two driverless mobility innovations using distinct lifecycles. The paper presents an analysis of the autonomous vehicle (AV) innovation lifecycle. It introduces four dimensions alone which to measure AV innovation performance over time. Finally, it presents five requirements that will need to be addressed before the use of autonomous vehicles can scale for consumer transportation and logistics.
Introduction
The innovation lifecycle is characterized by four phases: Research, Growth, Scale, and Maturity. During the Research phase new technology is invented. The performance of this technology tends to be low. The Growth phase starts when companies introduce products that improve upon the original innovation by addressing stated or perceived market needs. Early adopters use these products. During the Scale phase more companies enter the market both to satisfy the growing demand, but also to introduce refinements that address additional market requirements. Adoption expands from the early to the late majority. Finally, during the Maturity phase the initial innovation and its follow-on refinements reach a steady state and are adopted even by laggards. During this period the goal of the companies participating in the market is to prolong their established business models by introducing the smallest number of sustaining innovations that will address the already developed market’s needs.
The AV Innovation Lifecycle
The autonomous vehicles innovation lifecycle started around 2004 with the announcement of DARPA’s first Grand Challenge. Google launched Project Chauffeur, its autonomous vehicle project, in 2009. By the middle of the current decade, venture capitalists (VCs) and corporations started investing in startups developing autonomous vehicle technologies. To date companies offering on-demand mobility services have raised globally $75B. Over 50 OEMs and Tier 1 suppliers around the world have established automated and autonomous vehicle programs. CB Insights reported that during first three quarters of 2018 VCs committed $4.2 billion in the sector, compared to $3 billion in 2017 and $167 million in 2014. Finally, AlixPartners reports that an additional $61B has been earmarked for AV technologies. To date six broad use cases for autonomous ground vehicles have been identified: specialized vehicles operating in controlled environments, low-speed autonomous passenger shuttles, robotaxis, privately-owned vehicles, long-haul trucks, and delivery vans, including sidewalk robots. As is shown in Figure 1, currently each case is at a slightly different position along the AV innovation lifecycle.

Figure 1: The position of the AV use cases along the innovation lifecycle
Specialized autonomous vehicles are the farthest along and are placed on the lifecycle’s Growth phase. They are already being used in mines to move ore, and in ports to handle containers. Waymo, May Mobility, and Starship Technologies have launched robotaxi, low-speed autonomous shuttle, and last-mile delivery pilots in certain geofenced urban areas. Over the 12-18 months low-speed autonomous shuttles and last-mile delivery vehicles are expected to exit the Research and enter the Growth phase. Waymo has been expanding its robotaxi pilots and in fact, has already placed initial vehicle orders for robotaxi fleets.
To measure the performance of autonomous vehicle innovation we use four dimensions: Trust, Vehicle Reliability, Business Model, and Regulation.
Trust
Trust measures a user’s certainty that the autonomous vehicle will transport passenger and/or goods to the intended destination safely (no crashes), efficiently (the vehicle will not take circuitous routes in order to avoid situations under which its systems may not perform well), and correctly (the vehicle will arrive at the specified pick-up location at the promised time, and deliver its cargo at the correct drop-off location). Given the important role of data in autonomous vehicles and mobility services, users will additionally need to be convinced that: a) only the data necessary for providing the desired mobility service will be collected by the service’s operator, b) the collected data and associated inferences will adhere to established regulations and will be properly safeguarded, and c) the data and the inferences won’t be used in a way that is nefarious and harmful. To a lesser degree, and particularly in car-centric societies like the American, trust may also measure the public’s willingness to cede transportation control to a robot.
Embedded in the notion of trust is that of safety, particularly in the case of people transportation (no harm will be done to the passengers being transported). Surveys about consumer intent to utilize AV transportation showed that passengersafety while riding in these vehicles is indeed a significant concern. Figure 2 shows results from research conducted by the Pew Center in 2017. The majority of those surveyed indicated that they wouldn’t want to ride in an autonomous vehicle. Of those, 30% cite safety concerns as the reason for their decision, a number that is almost double of those who indicated that they would want to ride in such vehicles because they consider them safer than human drivers. Moreover, 42% of those who wouldn’t ride in AVs cited lack of trust in such vehicles.

Figure 2: Pew Research survey results on consumer sentiment towards autonomous vehicle safety
Data from Deloitte’s most recent annual Global Automotive Consumer Study (Figure 3) shows that across different geographies about the same percent of surveyed consumers are concerned about AV passenger safety. Even though the numbers have improved over the past two years, the public remain very concerned about autonomous vehicle safety. Perhaps this is due to the accidents that occurred early in 2018, starting with Uber’s fatal accident. The Deloitte, and more recently the AAA, surveys show more negative results relating to consumer trust of autonomous vehicles than those presented by Pew Research.

Figure 3: Percent of consumers who agree that AVs won’t be safe (Deloitte automotive survey 2019)
It is necessary to distinguish between passenger safety relating to the operation of the vehicle’s AV Stack, i.e., the collection of hardware and software components that under a particular configuration enable the vehicle to move autonomously, and the vehicle’s crashworthiness. If due to the AV Stack’s faulty operation the autonomous vehicle is involved in a collision, then the Stack is unsafe regardless of whether passengers were unharmed because of the vehicle’s crashworthiness. For example, assume that a Hummer robotaxi and a Prius robotaxi use the same AV Stack and operate in the same urban environment. The Hummer robotaxi has higher crashworthiness than an autonomous Prius because the Hummer’s design, construction, weight, etc., provides better protection to its passenger than the Prius in the event of a typical urban vehicle crash. Therefore, the Hummer may be perceived as safer than the Prius even though their AV Stack is unsafe. Based on the existing light regulatory environment even autonomous low-speed passenger shuttles, such as those used by May Mobility and others, are non-compliant with vehicle safety regulations. RAND provides a detailed framework for assessing autonomous vehicle safety across the development, validation, and deployment phases of these vehicles.
Finally, besides passenger safety, autonomous vehicle technology developers and regulators will need to concurrently address issues relating to the safety of those operating outside such vehicles. Cities consider autonomous vehicle an important component to their Vision Zero planning because of the expectation that they will be better at adhering to traffic rules than human drivers. Important work has started on how autonomous vehicles can understand and react to pedestrian behavior, as well as how pedestrians, bicyclists, and drivers can interact with autonomous vehicles.
Vehicle Reliability
AV reliability measures the autonomous vehicle’s ability to accomplish three goals. First, to perform correctly in the prescribed operating design domain for the duration of each ride without requiring human intervention, implying that it will not experience disengagements that will require a human to take over the vehicle’s operation. Human intervention may exist in the form of backup drivers riding in the vehicle, as is the case today with the autonomous vehicles being tested by companies that want to offer mobility services. Alternatively, humans may be able to remotely take over the vehicle’s operation using systems such as those being developed by Phantom Auto. Second, to perform consistently at the autonomy level for which it is rated, under ratings that are defined by one or more regulatory bodies. For example, a vehicle that is rated with Level 4 driving automation must perform in exactly the same manner every time it encounters a particular situation. Third, to provide rides of quality that its passengers find acceptable. For example, today many passengers participating in robotaxi tests such as those conducted by Waymo, Aptiv, and other companies, complain that these vehicles often stop abruptly, or move slower than posted speed limits becoming a hazard to the traffic around them. Reliability is principally based on the performance of the vehicle’s AV Stack, but also on the transportation infrastructure where the vehicle operates, and the communication technology used by the vehicle to interact with its environment.
Business Model
The business model dimension measures the AV operator’s ability to create a scalable business that will, over time, result in sustainable profitability. Identifying scalable business models is a complex issue in any area. In autonomous vehicles consumer transportation will obviously employ different models than logistics, and more likely each of the six broad cases mentioned will employ one or more different business models. For example, companies offering consumer transportation services may need to consider several sub cases to the general robotaxi use case, such as robotaxis offering exclusively rides to and from major city airports. Such companies may create a scalable business model that combines price/mile with in-vehicle advertising. By offering only one type of ride, using AVs that are expected to have lower cost/mile than human-driven vehicles, and combining distance-based revenue with advertising revenue, the mobility service’s operator may be able to charge lower prices and still show a higher profit than a competitor that offers a variety of ride types and relies exclusively on distance-based revenue. Similar analyses can be performed for other sub cases, e.g., daily commute rides from suburbs to downtown, and the right business models identified.
RegulationR
Regulation measures the extent and appropriateness of available laws and processes that govern the operation of autonomous vehicles and the fleets that use them. Regulation has to address a) the vehicle’s AV Stack, b) the data that makes that operation possible (infrastructure data, vehicle-generated data, fleet operation and management data, data coming from the various content providers participating in autonomous mobility), and c) the data that is generated by the users of the transportation and logistics services. Regulation is primarily addressed through advocacy and lobbying, with a certain level of trust and reliability being prerequisites. Regulation relating to autonomous vehicles will not be an exception.
Figure 4 shows a qualitative measure of the autonomous vehicle innovation performance in terms of the four identified dimensions. It presents where we are today along these dimensions, and where we need to be in order to reach the lifecycle’s Scale phase.

Figure 4: Four dimensions characterizing autonomous vehicle innovation performance
Using the position assigned to each of the six AV use cases along the innovation lifecycle (Figure 1), Figure 4 depicts an “average” position for the entire autonomous vehicle field. At present we don’t view that the logistics-related use cases, taken together, should be in a different position than the passenger transportation-related use cases. Because AV technology has improved dramatically over the past 10+ years, vehicle reliability is ahead of every other dimension, with regulation being the farthest behind. The position along the trust dimension is based on the data presented earlier in this paper. Companies offering consumer transportation and/or logistics services using autonomous vehicles have just started experimenting with business models. It is too early to tell whether any of these models will prove enduring and scalable. And even though some regulation has started at the state level, regulation related to autonomous vehicles is the dimension where we are farthest behind.
Five Requirements for Growing and Scaling Autonomous Mobility
I am proposing five requirements that will need to be satisfied in order to move autonomous vehicles from their current position on the innovation lifecycle to the Growth and ultimately the Scale phase. Satisfying these requirements will improve the performance along all four of the identified dimensions and result in the end state shown in Figure 4.
Requirement 1 (Continued investment is necessary to achieve the right reliability): Aggressive investment in autonomous vehicle technology development, and in infrastructure (transportation, communication, electrification) development must continue even if the timeline to the anticipated ROI may be longer than originally envisioned. The public is starting to realize that developing autonomous vehicles that can operate under any weather condition, in unconstrained rather than small geofenced areas, and on transportation infrastructures of any quality is much harder to achieve than certain technology developers, business executives and the media had us believe. Even though we have achieved 90%+ of the necessary functionality, the last 10% may actually require 90% of the overall effort. Because of their magnitude, future investments will not come from VCs but from private equity investors, e.g., Softbank’s Vision Fund, sovereign funds whose countries consider autonomous mobility a national imperative, e.g., Singapore’s Temasek, or corporations and corporate consortia, e.g., see Honda’s investment in GM’s Cruise unit.
It is also necessary to develop technologies for managing the vast amounts of data being captured from autonomous vehicles and the environments and infrastructures where they operate. Finally, it is imperative that we continue to develop new AI technologies that utilize new hardware architectures and massive parallelism for the analysis and exploitation of this data. Alongside, establish regulations for safeguarding data privacy and ensuring cybersecurity.
Requirement 2 (Test broadly and iterate fast to identify scalable business models): Accelerate the wider deployment of the use cases that can be employed safely with the available autonomous vehicle technology in the broadest operating design domains possible. Use these deployments to test and iterate quickly through a variety of business models. In particular, low-speed autonomous shuttles, and sidewalk robots used for last-mile deliveries are two of the use cases that can be deployed safely more broadly than they are today. We can start to quickly test a variety of business models for these use cases in various geographies and markets. For example, by partnering with cities that have average, or below average, public transportation networks, autonomous shuttles can be used to provide better overall service under a single ticket price. Medium size grocery chains can use sidewalk robots to provide free next-hour delivery of orders above a specific dollar value in order to win new customers in certain market segments.
Requirement 3 (Develop user trust through extensive testing): Autonomous vehicles incorporate many AI-based subsystems. Several of these utilize statistical machine learning and their behavior is not always deterministic. Consumers are starting to understand this even if they don’t fully comprehend what it means. Trust towards AVs will not develop only by placing human drivers in autonomous vehicles for some initial period. Companies that are developing such vehicles will need to work with regulatory bodies to convince the users of such vehicles that all their subsystems have been thoroughly tested with the test results validated and certified, very much like we do today to certify vehicle crashworthiness, airplane certification. This approach will need to be coupled with extreme transparency and may require the development of new testing techniques and processes that will be considerably different from the one used for the testing and validation of the software systems found in today’s conventional vehicles.
Requirement 4 (Educate the public and other interested parties): Companies and governments involved in next-generation consumer mobility must embark on various forms of education aimed at transforming the public’s anxiety to excitement about and comfort with riding in or otherwise using autonomous vehicles. Similar education will be necessary for truck drivers who are currently concerned that autonomous trucks will result in significant job losses even though the demand for truck drivers cannot be filled. Automated and autonomous logistics vehicles must be positioned as providing safety enhancements, increase the quality of the driving experience, and improve productivity. Improving trust, particularly for consumer transportation, will require various forms of education coupled with advertising campaigns. PAVE is an attempt to do this.
Requirement 5 (Start work to create the appropriate regulation): Creating the appropriate regulations that will provide the right controls without stymieing growth (consider the impact on Uber’s growth when London restricted the company’s operations) should become a high priority. Policy-makers must accelerate their efforts in order to catch up with the progress we have made on technology and reliability, and the corresponding progress we are making on business models. But in the process regulators must develop a good understanding of the AV technology, as well as the data and AI technologies being utilized by such vehicles and on-demand mobility services.
Autonomous mobility continues to generate excitement and deservedly so. It has the potential to change urban landscapes, make transportation safer, and supply chains faster. But in many instances its introduction will need to reverse decades long practices and habits. Creating enduring businesses based on this technology under such conditions requires a lot more than developing the technology itself. Understanding a target market segment’s requirements and concerns, selecting the right use cases that can satisfy these so that we can begin our business-building efforts, testing and rapidly iterating through candidate business models appropriate for the selected use case, and establishing the criteria that must be met before we begin large deployments are extremely important steps. Today we continue to focus too much on technology development while avoiding to launch similar efforts to address the other requirements of building sustainable businesses. By understanding what impacts the performance of autonomous vehicle innovation and what requirements will need to be addressed in order to move from the Research phase to the Growth and Scale phases we should be able to generate many exciting businesses and realize autonomous mobility’s full potential.
The previous article in the series.
Pingback: The Fuse | Crosspost: Six Requirements for Growing and Scaling Autonomous Mobility - The Fuse
Pingback: Seven Implications of Multimodal And Shared On-Demand Mobility – Re-Imagining Corporate Innovation with a Silicon Valley Perspective